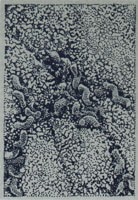
At issue is whether a patient with peptic ulcer disease (PUD) should receive empiric treatment for HP, or whether "confirmatory" testing is indicated. Early estimates suggested that 95% of patients with PUD will have HP (I recall that figure from the 13th edition of Harrison's Principles of Internal Medicine, now in it's 18th edition), but three epidemiological studies in the 1990s challenged this estimate as too high, with new estimates of 73% (study n=2394), 61% (study n=144), and 36% (studied n=95). The binomial confidence intervals (CIs) for those numbers are 71-75%, 53-69%, and 26-45%. The first study, with an estimate of 73% and n=2394 is actually a summary statistic derived from a combination of six other studies. So, which estimate do we choose? We could do a meta-analysis and combine all of these studies, but we already know what the result will be becasue the first study will trounce the others when weights are assigned. So I'm going to just conclude that while the prevalence of HP in PUD might not be 95%, it is likely close to 73%.
This is important because you have to decide what threshold of probability you will use to trigger treatment of HP. If that threshold is 75%, well then you might as well just treat everybody with PUD because the prior probability is so close to your treatment threshold. One of four of the patients you treat will have been treated "unnecessarily", on average. If that threshold is too low, then you need to raise it with testing. But, testing is not without its own set of pitfalls, and the gains in reducing false positives may be much less than you think.